Dr. Mun has been invited to be a Guest editor of the Special Issue Machine Learning for Computer-Aided Diagnosis in Biomedical Imaging in the Open-Access Journal, Diagnostics (ISSN 2075)
April 18, 2021
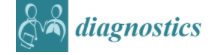
Special Issue "Machine Learning for Computer-Aided Diagnosis in Biomedical Imaging"
A special issue of Diagnostics (ISSN 2075-4418). This special issue belongs to the section "Machine Learning and Artificial Intelligence in Diagnostics". More info here:
https://www.mdpi.com/journal/diagnostics/special_issues/Computer-Aided_Diagnosis
Deadline for manuscript submissions: 30 November 2021.
Prof. Dr. Seong K. Mun Guest Editor
Arlington Innovation Center: Health Research, Virginia Tech -NCR, 900 N. Glebe Road, Arlington, VA 22203, USA
Interests: Radiology; Diagnostic Radiography; Biomedical Imaging; Medical Imaging; Biomedical Signal Processing; Medical Image Analysis; Neuroimaging; Diagnostic Imaging; Biomedical Engineering; Biomedical Instrumentation
Prof. Dr. Dow-Mu Koh
Guest Editor
Department of Diagnostic Radiology, The Royal Marsden NHS Foundation Trust, London, UK
Interests: Diagnostic Radiology; Oncological Imaging; Functional Cancer Imaging; Body diffusion-weighted MRI, Whole body MRI, Hepatobiliary and pancreatic cancers, Malignant bone disease, Radiomics and artificial intelligence
Special Issue Information
Dear Colleagues,
The radiology imaging community has been very active in developing computer-aided diagnosis (CAD) tools since the early 1990s, before the imagination of artificial intelligence (AI) fueled many unbound expectations in medicine as well as in other industries. Today, there are more than 50 FDA-approved AI imaging products in the US, but clinical adoption of these products has been very slow. However, the successful adoption of some AI imaging tools indicates that AI can positively impact medical imaging services. This Special Issue plans to focus on topics and issues to make AI more meaningfully intelligent for radiology and pathology. Imaging diagnostic services are far more than just radiologists or pathologists making a diagnosis. Imaging workflow is a very complex and labor-intensive process, often under stressful situations. CAD research in the past focused on diagnosis processes only. AI tools will have to look at the entire workflow beyond diagnosis. In AI development, we have seen success in laboratories that did not translate to real-world successes. The root causes of such failures have spurred the development of better science beyond traditional convolutional networks (CNN). There are better tools to manage the poor quality of input data and insufficient data volume for training and testing. Experience in other industries shows us that using various deep learning tools—supervised, unsupervised, and others—can address these complex issues associated with workflow and productivity improvement. AI is also being applied to image acquisition to improve image quality or accelerate acquisitions, thereby enhancing diagnostic performance while shortening examination times. Recent advances in quantitative imaging interns of radiomics and pathomics offer new tools for more integrated diagnosis, especially between pathology and radiology. In short, through this Special Issue, we would like to bring forth next-generation AI theories, tools, and solutions toward radiology and pathology imaging that can ultimately dramatically improve patient care.